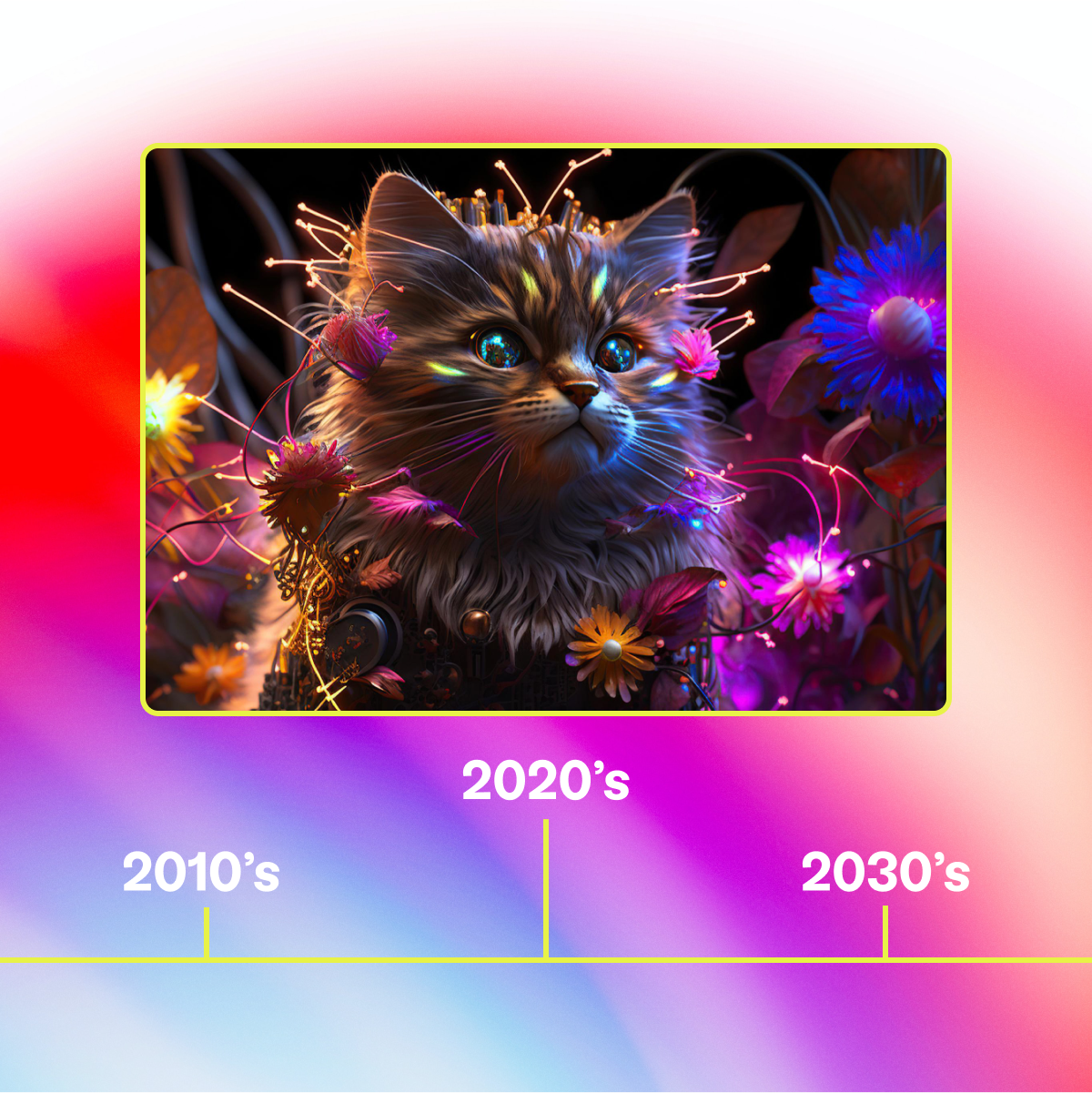
Generative AI is all the buzz at the moment. While traditional AI systems are like robots that follow instructions and can only do what they’ve been explicitly taught, generative AI is like a curious child, constantly learning and exploring. It can create new things by learning from the data it is given.
According to the latest McKinsey Global Survey on the state of AI, a whopping 60% of organizations that have reported AI adoption are using generative AI. It’s no wonder McKinsey has dubbed 2023 as "generative AI's breakout year." But here’s the thing — generative AI has been around for quite some time, evolving through several fascinating phases in the background. Below, we delve into the fascinating journey of generative AI, from its humble beginnings in the 1950s to its current prominence in the creative industry. Let’s get into it!
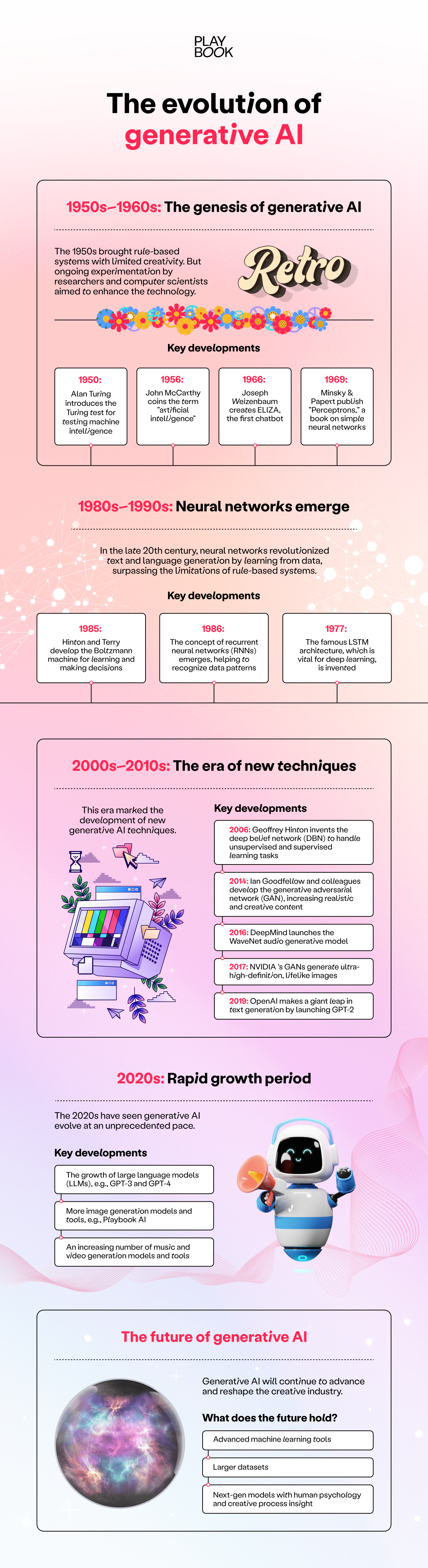
The genesis of generative AI (1950s–1960s)
The history of generative AI dates back to the mid-20th century, specifically the 1950s and 1960s when the groundwork for creative generative AI was laid. During this era, natural language processing (NLP) research primarily focused on rule-based systems, which relied on explicit programming to generate content. Unfortunately, this approach inherently limited the systems’ creative capabilities.
It was during this period that computer scientists and researchers began experimenting with rule-based systems, language generation, and the concept of using learning algorithms to generate new data. One of the most notable early successes was ELIZA, a chatbot designed to simulate a conversation with a therapist. While rudimentary by today's standards, the chatbot was a pioneering step in the world of generative AI.
Here are some of the key milestones from the 1950s and 60s:
- 1950: Alan Turing published his paper, "Computing Machinery and Intelligence," which introduced the Turing test, a test of a machine's ability to exhibit intelligent behavior equivalent to, or indistinguishable from, that of a human.
- 1956: John McCarthy “the father of AI” coined the term "artificial intelligence" at a conference at Dartmouth College.
- 1966: Joseph Weizenbaum created ELIZA, the very first chatbot.
- 1969: Marvin Minsky and Seymour Papert published “Perceptrons,” a book that introduced and analyzed the ‘perceptron,’ a simple neural network that can be used to learn patterns.
The emergence of neural networks (late 20th century)
The 1980s brought a significant shift in generative AI with the development of artificial neural networks. Inspired by the human brain, these neural networks could learn from data in a way that rule-based systems couldn't, and this breakthrough opened the doors to more realistic and creative content generation.
In particular, recurrent neural networks (RNNs) played a pivotal role in sequence generation tasks like language modeling and text generation. These neural network architectures enabled AI systems to understand and generate human-like text and narratives by identifying patterns and predicting the next likely scenario.
Around the mid-1980s, Geoffrey Hinton (the “godfather” of AI) and Terry Sejnowski developed the Boltzmann machine, a type of artificial neural network that's used for learning and making decisions. The neural network resembles interconnected switches that learn from data to solve problems and make predictions, making Boltzmann machines valuable for tasks like pattern recognition, making recommendations, and solving optimization problems.
In the 1990s, a significant breakthrough occurred with the emergence of deep learning, a form of machine learning that leverages the capabilities of neural networks to gather insights from data. A pivotal moment arrived in the late 1990s when long short-term memory (LSTM) networks, which are integral to deep learning, were developed. Deep learning has been a driving force behind the recent leaps in generative AI, enabling the creation of even more realistic and creative content.
The 2000s to 2010s
The early 2000s marked the development of new generative AI techniques, such as deep belief networks (DBNs). These artificial neural networks could handle both unsupervised and supervised learning tasks, paving the way for more realistic and creative content generation. However, limitations still existed.
Then came the mid-2000s to late 2010s, which saw rapid growth in generative AI models. Notable advancements during this period included the development of variational autoencoders (VAEs) and generative adversarial networks (GANs). GANs, in particular, emerged as a formidable generative AI technique that remains powerful to this day.
GANs operate by pitting two neural networks against each other: the generator and the discriminator. The generator aims to create realistic content, while the discriminator's role is to distinguish between real and fake content. This competition drives both networks to continually improve, resulting in increasingly realistic and creative content.
In 2017, NVIDIA's progressive GANs took things to the next level, enabling the generation of ultra-high-definition, lifelike images. The addition of extra layers during training led to unparalleled realism and clarity in the images produced.
Additional noteworthy advancements in the 2010s include:
- DeepMind's WaveNet: Launched in 2016, this neural network marked a significant milestone in audio generative models, generating realistic-sounding human speech and enabling highly accurate text-to-speech synthesis.
- OpenAI's GPT-2: In 2019, OpenAI made a giant leap in text generation with GPT-2, allowing coherent and contextually relevant sentence generation.
The 2020s
The 2020s have seen generative AI evolve at an unprecedented pace, with growing interest in its applications across various creative fields. Some of the most notable advances in generative AI tools and models for creatives in recent years include:
The growth of large language models (LLMs)
One of the standout developments is the creation of large language models (LLMs) like GPT-3 and GPT-4. These models can generate text that is virtually indistinguishable from human-written content, and they've been used to craft realistic news articles, poetry, and even code, demonstrating their versatility.
LLMs have reached a point where they can generate text with exceptional quality and coherence, making them invaluable for content generation, storytelling, and even chatbots that mimic human interactions.
Image generation models
In the realm of visual creativity, models for generating images have taken center stage. StyleGAN, for instance, can create lifelike images of people with a variety of facial features and hairstyles. Adobe Firefly can generate realistic images from text descriptions, apply text effects, enhance images with AI-powered features, and generate variations of both images and text.
Moreover, Playbook AI has emerged as a versatile platform for creative professionals, enabling AI art generation through models like DALL-E and Stable Diffusion. The best part about using Playbook is that you can not only generate AI art, but also store and manage it (including the AI prompts) from a single interface.
Image generation models have found applications in everything from design and art to fashion and filmmaking. They even enable the creation of realistic visual effects for movies and TV shows, reduce the need for extensive photoshoots, and provide designers with an endless well of creative inspiration.
Music and video generation models
Generative AI has also made significant strides in music generation. The MuseNet deep neural network, for example, can compose original songs and melodies that sound exactly like human-composed music, opening up new possibilities for musicians and composers. Musicians can now experiment with AI-generated compositions, and AI can assist in the creation of background scores for films, video games, and advertisements.
Similarly, video generation models have not been left behind. Take, for example, Google's DeepMind AI. The technology can create realistic videos of people and objects in motion, making it useful in several areas, including animation, film, and virtual reality. AI-generated content can speed up the video generation process and reduce costs. Plus, it can be used for creating special effects, generating training videos, or even automating content creation for platforms like YouTube.
These advances are just the tip of the iceberg. The future of generative AI holds immense potential, with applications limited only by our imagination.
Getting ahead in your creative career with generative AI
Generative AI has come a long way from its rule-based origins in the 1950s to the AI powerhouses of today. Thanks to the development of new machine learning techniques, the availability of vast datasets, and the tireless efforts of researchers and engineers, generative AI has become an indispensable tool in the creative industry with many use cases.
The recent advances in generative AI are incredibly promising, and they are poised to continue reshaping the creative industry. Put another way, the future of creativity is here, and generative AI is leading the way.
At Playbook, we are committed to staying at the forefront of generative AI advancements. We've created a comprehensive e-book that dives deeper into the world of generative AI, exploring the latest trends and applications. That's why Playbook now comes with extensive AI support—from creating AI images to storing them in a safe, intuitive, and beautiful interface, so you can make AI work for you.